When people ask me what I do, I usually say something about exploring the edges of the market for intelligence and analytics capabilities, starting with social media data. I also like to connect threads from separate topics and look at things from unusual perspectives. With that as a warning of sorts, let's pull some threads from new methods, old metrics, and emerging science to see what they do together. It may sound like so much theory so far, but this is all about practical analytics for management.
Thread one: A new view of social media in a customer journey framework
It started with a briefing from SDL
on their new Customer Commitment Framework (CCF). I'm always interested to see people do something different with social media data, and I give bonus points for tools that provide quick and clear access to useful information.
SDL's approach is to monitor business performance at key points of customer journeys by analyzing what they have to say in social media. They want to know what people are thinking as they progress toward a decision, whether that decision is about buying a product, telling others, or becoming an advocate for the product. CCF's analysis is always presented in the context of a customer journey, so—in theory, at least—its numbers provide a drill-down into the performance of different parts of a company's marketing and operational performance as experienced by customers.
I haven't tried CCF and its dashboard component yet, but if it works as promised, its alignment to identifiable business levers could make it a valuable analytical tool.
Thread two: Exploring possible futures with agent-based modeling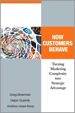
Call it simulation to avoid scaring people off, but the complexity science tool of agent-based modeling has come to market. When I saw that Icosystem had spun up a company, Concentric, to offer ABM tools for marketers, I knew I needed to learn about it.
Concentric's book, How Customers Behave, was a good start, but some of my earlier reading and the Santa Fe Institute's MOOC on complexity made the background sections somewhat redundant. One key takeaway I've found is that, despite the complexity label, this stuff isn't too complicated to understand.
Where SDL looks for signals about what has happened, Concentric starts by building models of customer journeys, playing out the decisions faced by individuals in the market ("agents"). Once the model can "predict" the past, it's ready for use in simulating the effects of different strategies and tactics. Depending on your needs, their software can incorporate social media and other online data sources, or it can look broadly across media types and operational data.
Is this what you expected?
If you put threads one and two together, you get simulations to explore possible outcomes of different strategies, and measurement of customer opinion at critical points to indicate actual performance. One looks forward to explore what may happen, and one looks at the recent past to understand what has happened.
It seems like a powerful combination to me, but let's add one more thread. What about hard data?
Thread three: Marketing analytics and the view of the process
I once worked on a project for one of the big phone companies that was concerned about customer churn in their high-speed Internet business. They were adding new customers as fast as they could, and they wanted to avoid losing existing customers. Our analysis rested on the insight that sometimes you lose the sale even when the customer wants to buy. Before it was trendy, we looked at the post-purchase customer journey and found some measurable issues.
I usually see customer journey models that assume that customer attempts to purchase virtually always succeed. If you're selling online or in retail, that's probably close to true. But do you know that it's true, or do you assume it?
For the phone companies, DSL service circa 2002 was constrained by geographic footprint, technical limitations, compatibility issues, and customer ability. Each of these added another step in the journey and another opportunity to lose the sale. Given the operational metrics they already had—order attempts, accepted orders, activations, etc.—you could track post-order performance as a series of multipliers between zero and one. A simple step-down chart would show you where you were losing customers, so you would know where to invest to improve the process.
For a subscription-based business, recurring revenue is everything, so you need to pay attention to customer retention and anything that drives them away. This is already a long post, so let me point to a post from Keith Schacht about customer acquisition and retention, and a VOZIQ
post on customer issues in telecom. The point is, your customer's experience may not end with the purchase, and your measurement of the experience shouldn't, either.
When you combine the effects of attracting new customers and losing existing customers, you end up with a survival analysis, which brings us back to complexity and the potential of agent-based modeling. The fact is, a given rate of customer additions and losses combine to set a ceiling on your possible customer base, so it's crucial to understand where and why you lose them.
Really, it all comes together
Pull these three threads together, and we start to see the potential of forcing analytics out of their comfortable silos. Agent-based models offer a tool for understanding how things might (not will) play out under different scenarios, including the possible outcomes of different marketing strategies. Analytics based on similar models of customer journeys provide a view of the recent past to test expectations and continually reevaluate the models. Combining social media data with customer data and operational metrics allows us to see a more complete picture: Where hard data is unavailable, social media indicators can fill the gaps. Where hard data is available, it provides a test of the social media indicators.
Pull the threads together, and you get a view that combines future and past; what people say and what they do; what happened and why. Sounds pretty useful to me.